
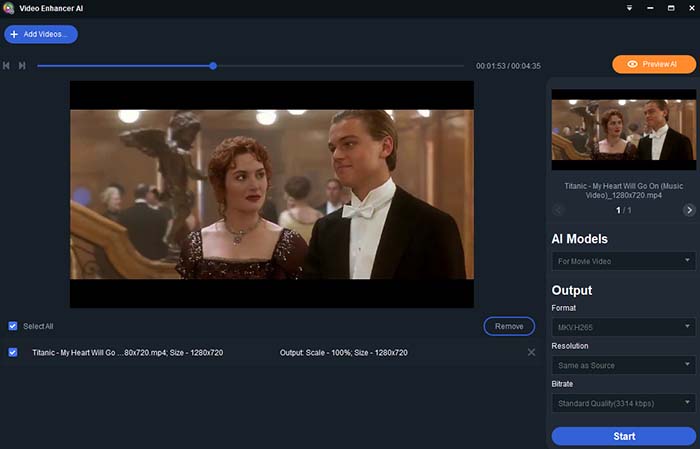
( C) Variant masking (setting both alleles as absent, represented by 0, corrupts data inputs at a gradually increasing masking rate). ( B) Ground truth whole genome sequencing data is encoded as binary values representing the presence (1) or absence (0) of the reference allele (blue) and alternative allele (red). Thus, the memory utilization for calculating correlations will be the same regardless of genomic density. For reducing computational complexity, we calculated the correlations in a fixed sliding box size of 500x500 common variants (MAF ≥ 0.5%). The red arrow illustrates minima between strong LD regions. ( A) Tiling of autoencoders across the genome is achieved by ( A.1) calculating a n x n matrix of pairwise SNP correlations, thresholding them at 0.45 (selected values are shown in red background, excluded values in gray), ( A.2) quantifying the overall local LD strength centered at each SNP by computing their local correlation box counts and splitting the genome into approximately independent segments by identifying local minima (recombination hotspots). Our autoencoder-based genotype imputation strategy achieved superior imputation accuracy across the allele-frequency spectrum and across genomes of diverse ancestry, while delivering at least fourfold faster inference run time relative to standard imputation tools.Īrtifitial intelligence autoencoder computational biology deep learning genetics genomics human imputation population genetics systems biology. Here, we demonstrate an autoencoder-based approach for genotype imputation, using a large, commonly used reference panel, and spanning the entirety of human chromosome 22.
FREE DENOISE PORTABLE
Artificial neural network-based imputation approaches may overcome these limitations by encoding complex genotype relationships in easily portable inference models.
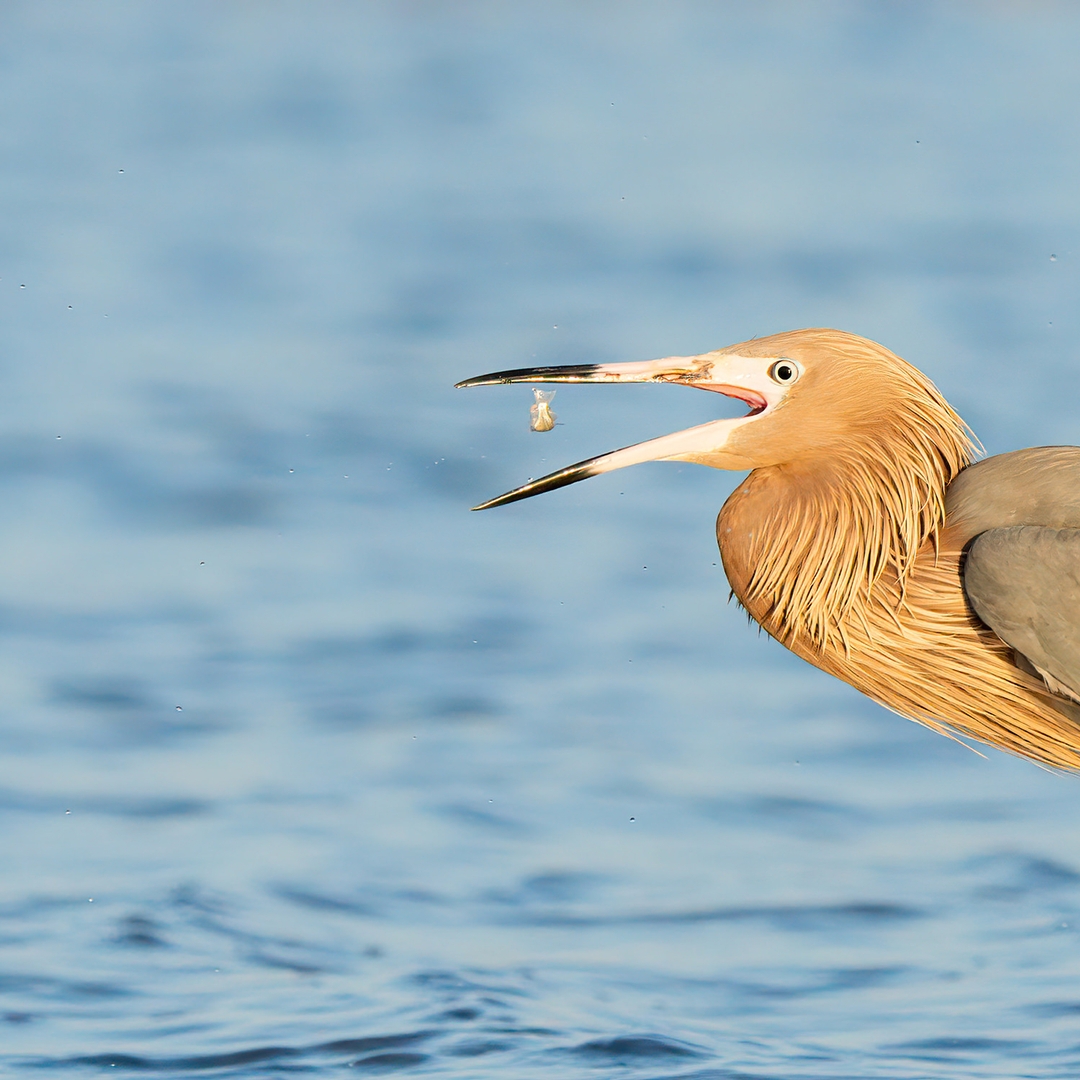
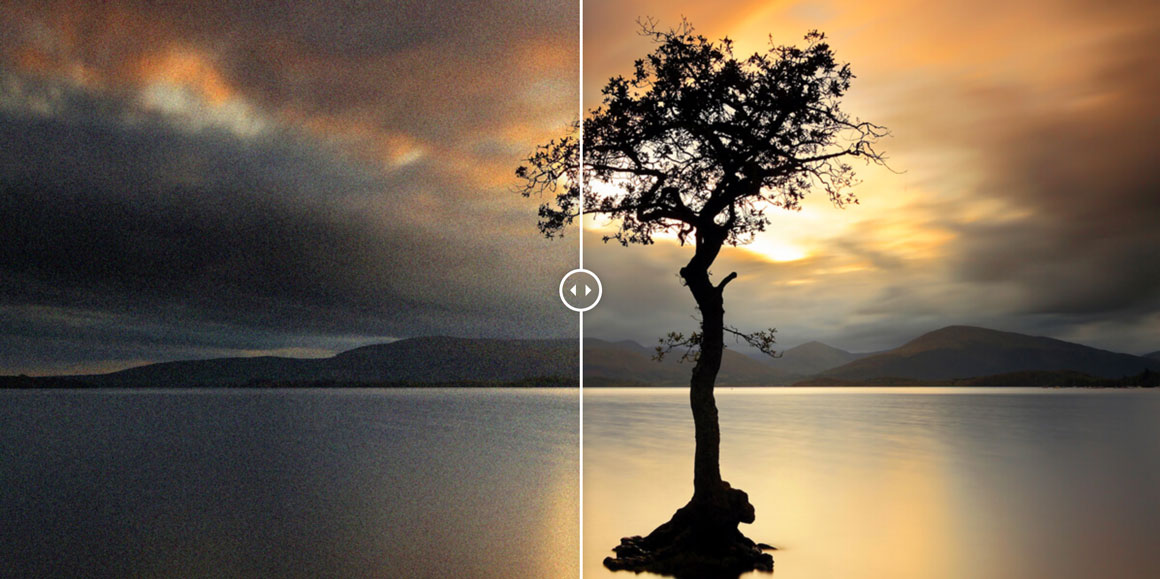
Moreover, the accuracy of current statistical approaches is known to degrade in regions of low and complex linkage disequilibrium. This results in computational resource and privacy-risk barriers to access to cutting-edge imputation techniques. Standard statistical imputation approaches rely on the co-location of large whole-genome sequencing-based reference panels, powerful computing environments, and potentially sensitive genetic study data. Genotype imputation is a foundational tool for population genetics.
